Summary:
By collecting relevant data, reviewing it in a critical manner, and creating a testable explanation, analyze confidently.
Why Quality User Tests are complicated to analyze
Data analysis from Quality Preventy Tests is often presented in textbooks, which, as a straightforward process of listing of use matters, summarizes work performance, describing mistakes, and summarizing time. However, it is not always so easy. Especially this is the case when you have:
- Unusual study design (such as a multiple prototype version shown to each partner in a session)
- How do people solve problems with complex research questions such as consequences, understanding, or people issues
- Fewer sincere prototypes that all features, content, or screens are not available
Let’s imagine that we have designed a new product deals page that we want to test. Participants will be asked to find specific products on a prototype and choose one of them which is best for them. The following are some of our research questions.
- Is Product Comparison features discovered?
- Which information about the product is important to users and what not?
- Do people understand product overview information?
- Do people understand how the product works? Do they create the right mental models?
It can be difficult to answer such research questions by studying performance in a task. We will need to answer these questions successfully:
- Collect multiple pieces of data (What did people do and said in several places in the session)
- Weigh the data against the design designDetails of the recruitment (who participated in our research), and even the events of the convenience (ie, the researcher did or said)
- Rectangle All this information to provide reliable answers
Analysis and synthesis
The data requires a combination of two activities to move towards insights and recommendations: analysis and synthesis.
Analysis refers to the breakdown and inspection of complex information, while the combination refers to the information recovery In new meaningful forms, ie insights. (Note that when we talk about “analysis” as a stage in the research process, we are really referring to these two activities.)
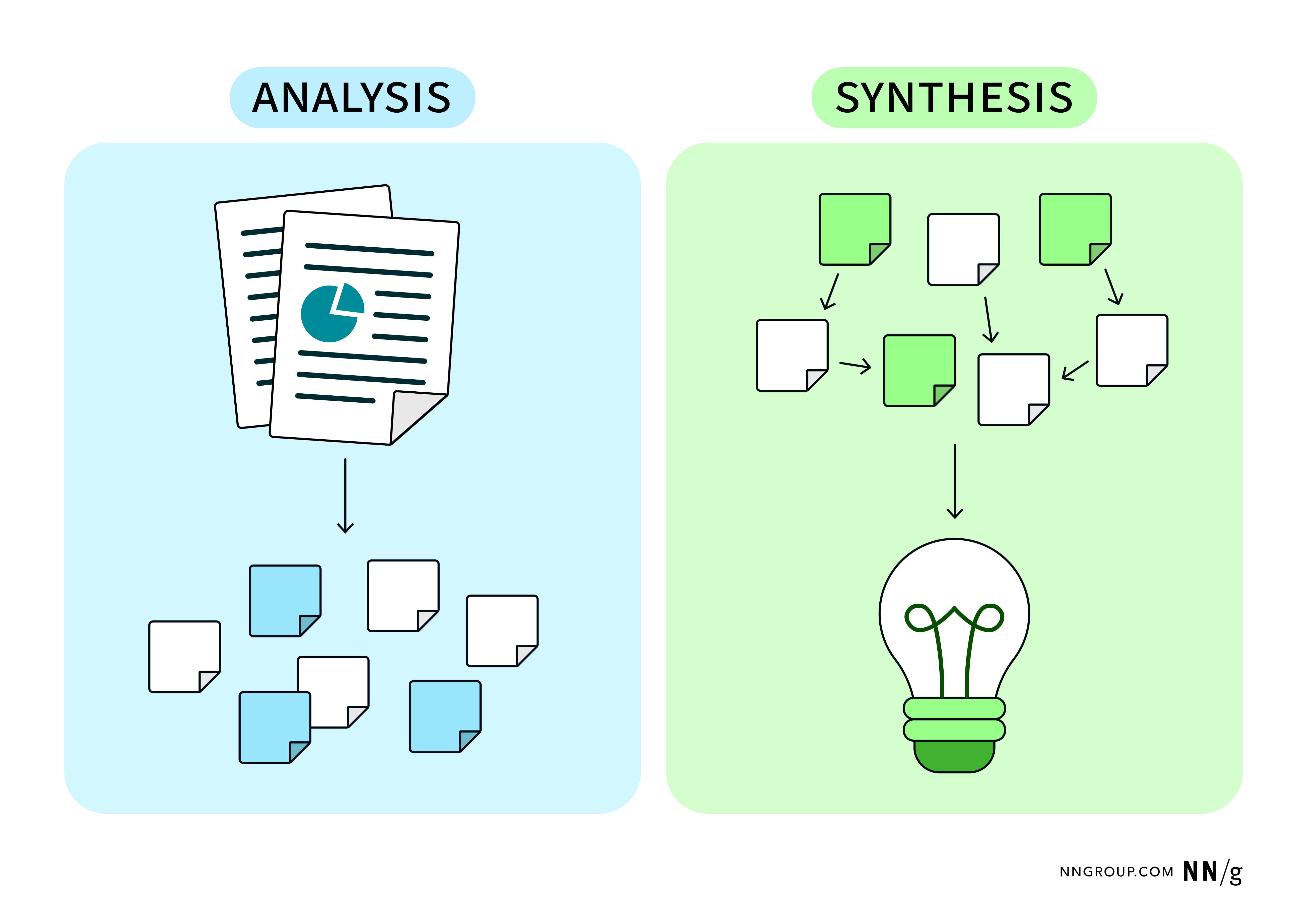
When we analyze the quarteto data, analysis and synthesis are not in clear linear fashion. Sometimes, we go back and forth.
4 steps to analyze use test data
To clarify how we reach out from the test data data and look like the process of analysis and synthesis in this context, we suggest 4 -step framework.
- Collect the relevant data: Select data points for each session (observations and pricing) that are related to the research question. The move involves to include analysis and reducing data.
- Evaluate accuracy: Test each data point to assess its compatibility and accuracy. We are still in the analysis phase.
- Specify the data: Data points (Step 1), our reviews (Step 2), and combine our skills to provide reasonable explanations or answers to our research question. The move includes the recipe.
- Check for good fit: Ensure good fit we check the answers or answers to our research questions against the data submitted. Does whatever data we have collected supports? If not, we reiterate the explanation (by reviewing Step 3). Step 4 is the place where the analysis is again.
Although this process seems linear, 3 and 4 steps are often repetitive. In practice, we create preliminary specifications based on a small subset of data – often data points that are easy to remember or easy to stand. However, when we examine these explanations against the wider dataset, we can look at contradictions or neglected patterns that challenge our initial thinking. This gives us an indication of improving our explanations. This can be several times ahead between 3 and 4 stages.
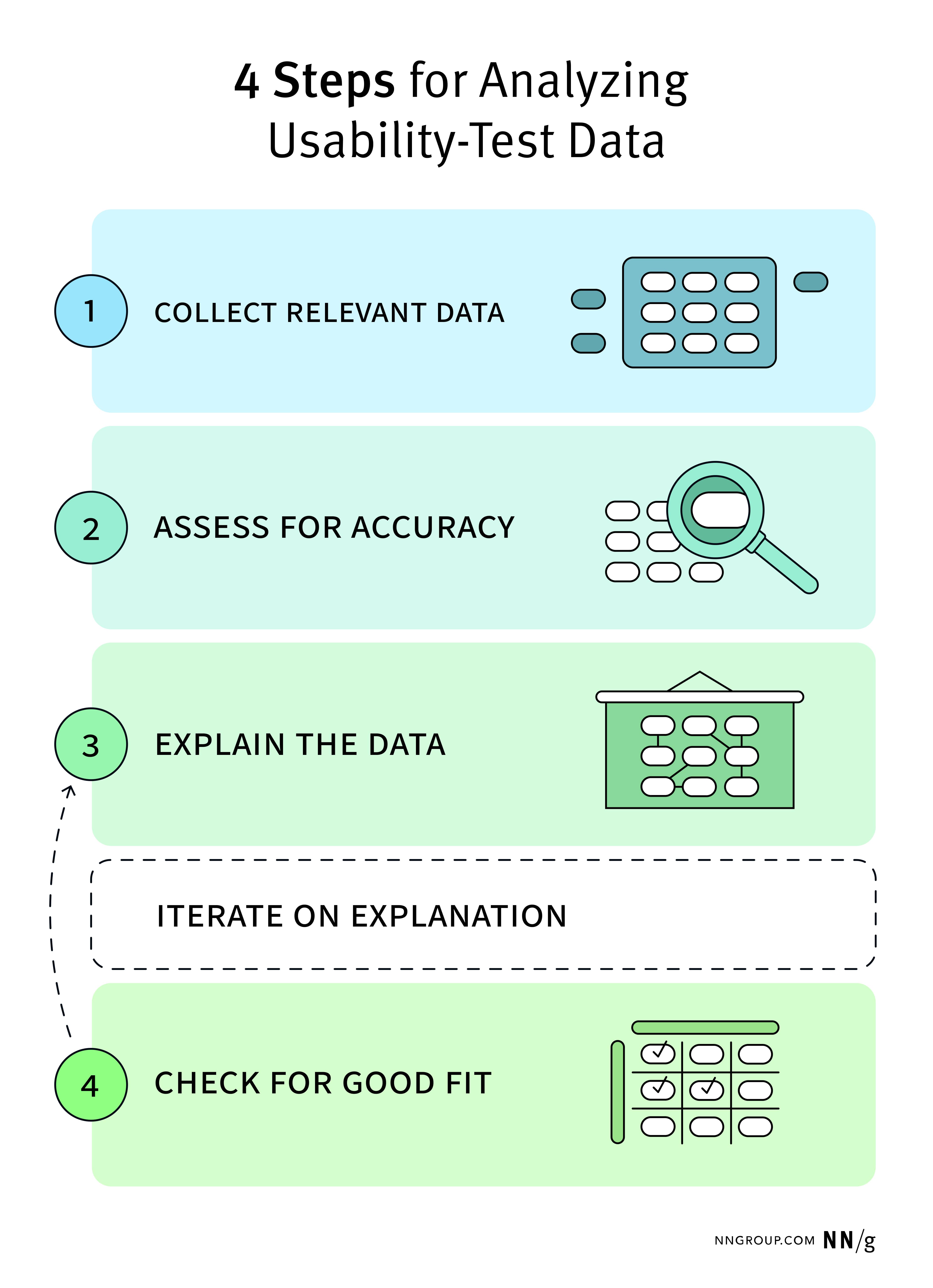
Step 1: Collect the relevant data
Usage tests produce many data. I Collect Stage, we start collecting all relevant data points or observations that can help us answer our research questions. (It’s a bit like picking up apples in a garden – we are choosing apples that look hopeful.) To do this, we inspect the session notes, transcripts and recording, if available. We enter relevant observations and prices.
For example, if we were looking for understanding whether the comparison feature on the product deals page is discovered, we are notices and records the facilitator’s follow -up questions while collecting data points on the participants’ behavior, momentarily comments, and performing related tasks. The questions we can ask are included:
- Did the participants use this feature or take notice of it (as indicated, for example, mouse cursor more than the feature)?
- Did the participants mention the need to compare in comparison features or in a loud voice or in response to some of the facilitator’s questions?
- If they did not use the comparison feature, how did the participants work?
The move includes analysis as we are breaking the full data set and extracting a small set of useful items.
Step 2: Evaluate accuracy
In Step 2, we are still in the analysis phase (instead of synthesis). I Evaluate Step, we check the data points to evaluate how much each one is relevant and how much weigh it on. Not every data point is treated equally. This is like an apple -choosing, that every apple selective inspects for every apple injury or other flaws.
For example, a partner may have commented that he likes the comparison feature but has never used it. Or perhaps this comment was in response to an important question of facilitator (such as, Do you like the comparison feature?), Knowing these details gives us a little or less confidence in the data point.
Step 3. Explain the data
In Step 3, we begin the process of synthesis by imposing our observations, diagnoses, and domain knowledge Generate potential specifications (Or fake concepts) for the data we have collected.
Sometimes we see the data collected, many specifications make it possible. For example, if all our participants have lost the comparison feature that we have designed, the following specifications can be created.
- Possible Explanation 1: This feature was not in the place where people were expected.
- Possible Explanation 2: It was difficult to see or notice the feature.
- Possible Explanation 3: This feature was not useful to the participants at the work, so they did not look for it.
To come with explanation, UX also requires knowledge and experience as well as imagination. For example, if a researcher has researched similar designs in the past, they may have many memories of human behavior and information about the best methods of design.
Step 4. Check for good fit
To reduce our potential specifications and to build confidence in our specifications about user behavior, we Check out our specifications against existing data For a good fit. Does the current data explanation support? Can we find data points that contradict it or can we cause us to doubt its accuracy? This step is like seeing whether a piece of puzzle fits clean.
For example, if the participants did not use the comparison feature on the product overview page and our interpretation was that consumers did not need it, we would expect that participants used another successful strategy to compare the product. However, if participants struggle to compare, many product overview pages in multiple tabs to compare, or complain that it is difficult to compare the available product, we can reject this explanation because it seems wrong in view of the data.
What’s going on here A version of assumption testing. Our explanation is predicted. If data does not support our predictions, we reject or improve our explanation.
Quality research often avoids valuable insights and answers – but can raise the same new questions that need more research! It is okay for your analysis to report that you do not know the reasons behind the data and need further research. However, the sign of a good analyst is detecting their data from several angles, examining numerous explanations against data, and pushing some sensible assumptions to discover in future research.
Conclusion
Analysis of data from quality prevailing testing is often more complicated than its imagery. This type of data is rich, proportional and dirty. A good analyst collects all the right data, evaluating it from multiple angles, finding an explanation that is in accordance with the data, and tests these explanations for a good fit.
Unlock Your Business Potential with Stan Jackowski Designs
At Stan Jackowski Designs, we bring your ideas to life with cutting-edge creativity and innovation. Whether you need a customized website, professional digital marketing strategies, or expert SEO services, we’ve got you covered! Our team ensures your business, ministry, or brand stands out with high-performing solutions tailored to your needs.
🚀 What We Offer:
- Web Development – High-converting, responsive, and optimized websites
- Stunning Design & UI/UX – Eye-catching visuals that enhance engagement
- Digital Marketing – Creative campaigns to boost your brand presence
- SEO Optimization – Increase visibility, traffic, and search rankings
- Ongoing Support – 24/7 assistance to keep your website running smoothly
🔹 Take your business to the next level! Explore our outstanding services today:
Stan Jackowski Services
📍 Located: South of Chicago
📞 Contact Us: https://www.stanjackowski.com/contact/
💡 Bonus: If you’re a ministry, church, or non-profit organization, we offer specialized solutions, including website setup, training, and consultation to empower your online presence. Book a FREE 1-hour consultation with Rev. Stanley F. Jackowski today!
🔥 Looking for a done-for-you autoblog website? We specialize in creating money-making autoblog websites that generate passive income on autopilot. Let us handle the technical details while you focus on growth!
📩 Let’s Build Something Amazing Together! Contact us now to get started.